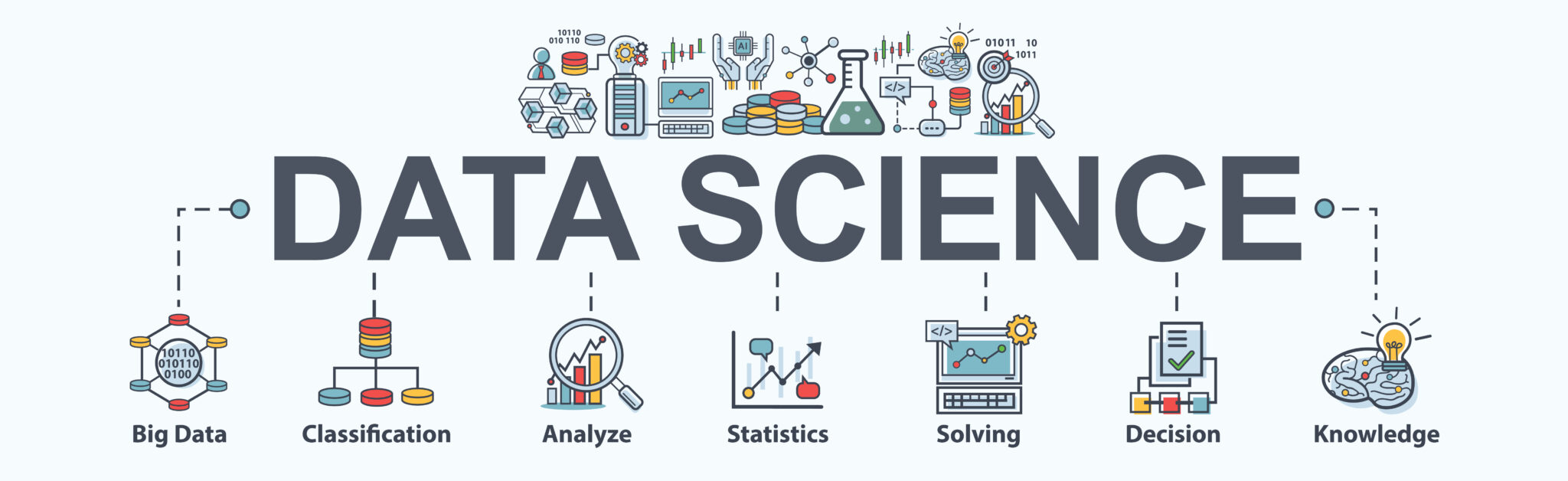
Details
The boom in digitization and modern technologies has caused a huge increase in data volumes. This data are typically characterized by heterogeneity, present temporal and/or spatial variations, while they contain different levels of correlations. In order to take advantage of their value and extract useful knowledge from them, we need to be able to properly store, filter and analyze them. Often, this data are stored in the format of data and/or knowledge graph structure, where the latter is a semantic network, representing a network of entities—i.e. objects, events, or concepts—and illustrating the relationship between them. Our research focuses on the development of combined model-based and data-driven frameworks that interplay between explainable ML/AI data analytics and graph-embedding models for improved data engineering and analysis, in order to enable scalable, efficient, accurate and usable analytics in diverse application domains and types of datasets.